Exam 3 Study Guide
The one-hour study guide for exam 3
Paul Krzyzanowski
April 2020
Last update: Wed Dec 13 16:49:54 EST 2023
Bigtable
Goal: Build a massively scalable table of data that is indexed by a row key, contains a potentially arbitrary and huge number of columns, and can be distributed among tens of thousands of computers.
Bigtable is a distributed storage system developed at Google that is structured as a large table: one that may be petabytes in size and distributed among tens of thousands of machines. It is designed and used for storing items such as billions of web pages indexed by URL, with many versions per page; hundreds of terabytes of satellite image data; or statistics and preferences of hundreds of millions of users. It is also designed to be able to handle thousands of queries a second.
To the user, an instance of Bigtable appears as a large spreadsheet: a table that is indexed by a row key, column name, and timestamp. Each cell can store multiple timestamped versions of data. If an application does not specify a timestamp, it will get the latest version. Alternatively, it can specify a timestamp and get the latest version that is no newer than that timestamp. All operations on rows are atomic but only on one row at a time. That is, a client cannot grab a lock for a set of rows in order to make changes.
Columns in the table are organized into column families. A column family is a related group of columns (e.g., “outgoing links” could be a column family). Each column family has a unique name and contains within it a list of named columns. A Bigtable instance will typically have a small number of column families (perhaps a few hundred at most) but each column family may have a huge number (perhaps millions) of columns within it. Bigtable tables are generally sparse. If a cell (a column in a row) is empty, it will not use up any space. Column families are configured when the table is created and are common to all rows of the table. Columns within a column family may be specific to a row and can be added dynamically to any row. An example of columns within a column family is a list of all the websites you visited (i.e., many thousands of columns, with a new one added when you visit a new website). The set of columns used between one row and another may be wildly different (which is what makes tables typically sparse). Each cell may contain multiple versions of data. Timestamped versioning is configured on a per-column-family basis.
A Bigtable table starts off as a single table. As rows are added, the table is always kept sorted by row key. As the table grows, it may split along row boundaries into sub-tables, called tablets. Tablet servers are responsible for serving data for tablets. If a tablet gets too many queries, Bigtable can balance the workload by splitting the tablet and moving one of the new tablets to a different server.
Bigtable comprises a client library (linked with the user’s code), a master server that coordinates activity, and many tablet servers. Each tablet server is responsible for managing multiple tablets. Depending on the size of the tablets, it may manage from tens to thousands of tablets. Tablet servers can be added or removed dynamically. Tablet data is stored within GFS (Google File System) and tablet servers run on the same machines as GFS chunkservers. A tablet server handles read/write requests for its tablets and splits tablets when they grow large.
New data to Bigtable is stored on the tablet server in a memory structure called a memtable. Once the size of the memtable gets larger than some threshold value, it is frozen and converted to an SSTable. The SSTable (sorted string table) is the file format used to store Bigtable data, managing the mapping of keys to their values. An SSTable is immutable. Any changes result in a new table and old tables will get deleted.
The master assigns tablets to tablet servers, balances tablet server load, and handles schema changes (table and column family creations). It tries to run the tablet server on the same GFS chunkserver that holds data for that tablet. The master is also responsible for garbage collection of unneeded files (e.g., old SSTables in a tablet) and managing schema changes (table and column family creation).
In Bigtable, Chubby is used to ensure there is only one active master, store the bootstrap location of Bigtable data, discover tablet servers, store Bigtable schema information, and store access control lists. Chubby stores the location of the root tablet, which is the top level of the three-layer-deep balanced tree. The top two layers of the tree are metadata tablets, which do not contain table data but only information to locate the tablet that is responsible for a particular range of key values.
Bigtable relies on GFS for protecting its data from loss but can be configured for replication to multiple Bigtable clusters in different data centers to ensure availability. Data propagation for this replication is asynchronous and results in an eventually consistent model.
Cassandra NoSQL database
Goal: Create a distributed NoSQL database system designed for high availability, fault tolerance, and efficient storage and retrieval of large volumes of data across many commodity servers.
Cassandra is a distributed NoSQL database system that is designed to handle large volumes of data across many commodity servers while providing high availability, fault tolerance, and low latency. Cassandra’s architecture is based on the concept of a wide-column data store and distributed hash tables.
In a wide-column database (also known as a column-family or columnar database), data is organized into tables where the columns for each row can vary in quantity and type. This allows for flexible schema design and efficient storage and retrieval of data. Cassandra uses the Cassandra Query Language (CQL) to interact with table data.
Cassandra’s architecture is based on a peer-to-peer distributed system that uses a ring-based architecture to distribute data across nodes in a cluster. Each node in the cluster is responsible for storing a portion of the data, and nodes work together to provide a highly available and fault-tolerant system.
Cassandra uses a Chord-style distributed hash table (DHT) to manage the distribution of data across the cluster. A DHT is a distributed data structure that maps keys to values, allowing nodes to efficiently locate data stored on other nodes in the cluster. Cassandra’s DHT is called the partitioner, and it uses consistent hashing to evenly distribute data across nodes in the cluster. This ensures that each node is responsible for a balanced and manageable portion of the data.
A row within a table is indexed by a primary key. The primary key in Cassandra may be composed of a combination of a partition key and one or more clustering keys (columns). The partition key determines which partition the data belongs to. This value is hashed and used by the DHT to locate the node responsible for that partition.
A clustering key is used to define the sequence of data within a partition. The clustering key is crucial for sorting and organizing data inside a partition, making it easier to retrieve and query data efficiently. When multiple clustering columns are used, they are combined in the order they are defined, creating a hierarchical structure to sort the data.
Cassandra’s replication feature provides fault tolerance by replicating data across multiple nodes in the cluster. Each data item in Cassandra is replicated across a configurable number of nodes, known as the replication factor. This ensures that if a node fails, the data can still be accessed from other nodes in the cluster.
Cassandra also provides tunable consistency, which allows developers to balance data consistency and availability according to their needs. Consistency can be tuned on a per-query basis, allowing developers to prioritize consistency for critical data while relaxing consistency requirements for less critical data.
Cassandra’s architecture also includes a distributed architecture for reads and writes. When a write request is sent to Cassandra, it is first written to a commit log on the node that received the request. The data is then written to an in-memory data structure called a memtable, which is periodically flushed to disk as a new SSTable file. Reads in Cassandra are typically served from the memtable, with the SSTable files being used to back up the data.
Spanner
Goal: Design a huge-scale worldwide database that provides ACID semantics, read-free locking, and external consistency.
Introduction
Brewer’s CAP theorem led to the popularity of an eventual consistency model rather than relying on transactional ACID semantics.
In this model, writes propagate through the system so that all replicated copies of data will eventually be consistent. Prior to the completion of all updates, processes may access old versions of the data if they are reading from a replica that has not been updated yet.
With ACID semantics, this would not happen since the transaction would grab a lock on all the replicas prior to updating them. Eventual consistency is the trade-off in designing a system that is both highly available and can survive partitioning.
The presence of network partitions is, in many environments, a rare event. By using an eventual consistency model, we choose to give up on “C” (consistency) in order to gain availability on the slim chance that the network becomes partitioned.
Given that partitions are rare in places such as Google data centers, which uses redundant networks both within and outside each center, it is not unreasonable to enforce a strong consistency model (which requires mutual exclusion via locks). Network partitions will rarely affect the entire system but only a subset of computers. As such, it is possible to use a majority consensus algorithm, such as Paxos, to continue operations as long as the majority of replicas are functioning. Spanner takes advantage of an environment where partitions are rare and is willing to be partially unavailable in that rare event when a network becomes partitioned.
Experience with eventual consistency has taught us that this model places a greater burden on the programmer. With an eventual consistency model, it is now up to the programmer to reconcile the possibility that some data that is being accessed may be stale while other data might be current. Bigtable, for example, was difficult to use in applications that required strong consistency. Custom code had to be written to coordinate locks for any changes that spanned multiple rows.
With Spanner, Google built a transactional (ACID) database that spans many systems and widely distributed data centers. Spanner provides the user with a large-scale database that comprises multiple tables, with each table containing multiple rows and columns. The model is semi-relational: each table is restricted to having a single primary key. Unlike Bigtable, transactions can span multiple rows and across multiple tables. Also unlike Bigtable, which provided eventually consistent replication, Spanner offers synchronous replication, ACID semantics, and lock-free reads.
Data storage
Spanner gives the user the the ability to manage multiple tables, each with rows and columns. Internally, the data is stored as a large keyspace that is sharded across multiple servers. Like Bigtable, each shard stores a group of consecutive of rows, called a tablet. This sharding is invisible to applications.
Tablets are replicated synchronously using Paxos. One of the replicas is elected to be a leader and runs a transaction manager. Any transactions that span multiple shards use the two-phase commit protocol. Replication is performed within a transaction and all replicas remain locked until replication is complete, ensuring a consistent view of the database.
Applications can specify geographic data placement and the amount of replication:
Proximity of data to users (impacts read latency)
Proximity of replicas to each other (impacts write latency)
Amount of replication (impacts availability and read performance)
Spanner was designed for a global scale and a database will span multiple data centers around the globe. In a data center, spanservers store tablets. A zonemaster periodically rebalances tablets across servers to balance load. The zonemaster does not participate in transactions.
Transactions
Spanner provides transactions with ACID semantics. Transactions are serialized to satisfy the “I” (isolated) property and to create the illusion that one transaction happens after another. Strict two-phase locking is used to accomplish this.
Transactions can be distributed among multiple systems (which may span multiple datacenters). Each transaction is assigned a globally-meaningful timestamp and these timestamps reflect the serialization order of the transactions. Once a transaction has acquired all the locks it needs, it does its work and then picks a commit timestamp. Two-phase locking can reduce overall perfomance because other transactions may need to wait for locks to be released before they can access resources. Spanner uses separate read and write locks to achieve greater concurrency but even these can often block another transaction. A read lock will cause any writers to block and a write lock will cause any other writers or readers to block.
Spanner also offers lock-free reads. For this, spanner implements a form of multiversion concurrency control by storing multiple timestamped versions of data. A transaction can read data from a snapshot, an earlier point in time, without getting a lock. This is particularly useful for long-running transactions that read that many rows of the database. Any other ongoing transactions that modify that data will not affect the data that the long-running transaction reads since those will be later versions.
External consistency
Serialization (isolation, the I in ACID) simply requires that transactions behave as if they executed in some serial order. Spanner implements a stronger consistency model, external consistency, which means that the order of transactions reflects their true time order. Specifically, if a transaction T1 commits before a transaction T2 starts, based on physical (also called “wall clock”) time, then the serial ordering of commits should reflect that and T1 must get a smaller timestamp than T2. Spanner does this by using physical timestamps.
It is not possible to get a completely accurate real-world timestamp. Even if we had an accurate time source, there would be synchronization delays that would add a level of uncertainty. The problem is compounded by having the database span data centers around the globe. Spanner tries to minimize the uncertainty in getting a timestamp and make it explicit.
Implementing external consistency
Every datacenter where spanner is used is equipped with one or more highly accurate time servers, called time masters (a combination of a GPS receivers and atomic clocks is used). The time master ensures that the uncertainty of a timestamp can be strongly bounded regardless of where a server resides. Each spanserver has access to a TrueTime API that provides a narrow time interval that contains the current time, ranging from TT.now().earliest up to TT.now().latest. The earliest time is guaranteed to be in the past and the latest is guaranteed to be a timestamp in the future when the function was called. These values would typically be only milliseconds apart. Spanner can now use these timestamps to ensure that transactions satisfy the demands of external consistency.
The key to providing external consistency is to ensure that any data committed by the transaction will not be visible until after the transaction’s timestamp. This means that even other systems that have a different clock should still see a wall clock time that is later than the transaction’s timestamp. To do this, spanner waits out any uncertainty. Before a transaction commits, it acquires a commit timestamp:
t = TT.now().latest
This is the latest possible value of the true time across all servers in the system. It then makes sure that no locks will be released until that time is definitely in the past. This means waiting until the earliest possible current time on any system is greater than the transaction timestamp:
TT.now().earliest > t
This wait is called a commit wait and ensures that any newer transaction that grabs any of the same resources will definitely get a later timestamp.
Note that there is no issue if multiple transactions have the same timestamp. Timestamps are strictly used for versioning to ensure that we provide a consistent view of the database while supporting lock-free reads. Consider the case of a transaction that needs to read hundreds of millions of rows of data. Many transactions may modify the database since you start your work but the view of the database will be consistent since all data read will be no later than a specified timestamp.
Summary
By making timestamp uncertainty explicit, Spanner could implement a commit wait operation that can wait out the inaccuracy of a timestamp and provide external consistency along with full ACID semantics. Coupled with storing multiple versions of data, Spanner provides lock-free reads.
Spanner’s design was a conscious decision to not sacrifice the strong ACID semantics of a database. Programming without ACID requires a lot of extra thinking about the side effects of eventual consistency and careful programming if one wants to avoid it. Strict two-phase locking and a two-phase commit protocol are implemented within Spanner so programmers do not have to reinvent it.
MapReduce
Goal: Create a massively parallel software framework to make it easy to program classes of problems that use big data that can be parsed into (key, value) pairs. Each unique key is then processed with a list of all values that were found for it.
MapReduce is a framework for parallel computing. Programmers get a simple API and do not have to deal with issues of parallelization, remote execution, data distribution, load balancing, or fault tolerance. The framework makes it easy for one to use thousands of processors to process huge amounts of data (e.g., terabytes and petabytes). It is designed for problems that lend themselves to a map-reduce technique, which we will discuss. From a user’s perspective, there are two basic operations in MapReduce: Map and Reduce.
The Map function reads data and parses it into intermediate (key, value) pairs. When that is complete and all (key, value) sets are generated, the Reduce function is called once for each unique key that was generated by Map and is given that key along with a list of all values that were generated for that key as parameters. The keys are presented in sorted order.
The MapReduce client library creates a master process and many worker processes on the available, presumably large, set of machines. The master serves as a coordinator and is responsible for assigning roles to workers, keeping track of progress, and detecting failed workers. The set of inputs is broken up into chunks called shards. The master assigns map tasks to idle workers and gives each of them a unique shard to work on. The map worker invokes a user’s map function, which reads and parses the data in the shard and emits intermediate (key, value) results.
The intermediate (key, value) data is partitioned based on the key according to a partitioning function that determines which of R reduce workers will work on that key and its associated data. The default function is simply hash(key) mod R that [usually] distributes keys uniformly among R reduce workers but a user can replace it with a custom function. These partitioned results are stored in intermediate files on the map worker, grouped by key. All map workers must use the same partitioning function since the same set of keys must go to the same reduce workers. Map workers work in parallel and there is no need for any one to communicate with another. When all map workers are finished processing their inputs and generated their (key, value) data, they inform the master that they are complete.
We now need to get all values that share the same key to a single reduce worker. The process of moving the data to reduce workers is called shuffling. After that, each reduce worker sorts the (key, value) data so that all the values fot the same key can be combined into one list. This is the sorting phase.
The master dispatches reduce workers. Each reduce worker contacts all the map worker nodes with remote procedure calls to get the set of (key, value) data that was targeted for them. The combined data for each key is then merged to create a single sorted list of values for each key. The combined process of getting the data and sorting it my key is called shuffle and sort.
The user’s reduce function gets called once for each unique key. The reduce function is passed the key and the list of all values associated with that key. This reduce function writes its output to an output file, which the client can read once the MapReduce operation is complete.
The master periodically pings each worker for liveness. If no response is received within a time limit, then the master reschedules and restarts that worker’s task onto another worker.
Bulk Synchronous Parallel & Pregel
Goal: Create a software framework for fault tolerant, deadlock-free parallel processing. Then, adapt that to create an software framework makes it easy to operate on graphs on a massive scale.
Bulk Synchronous Parallel (BSP)
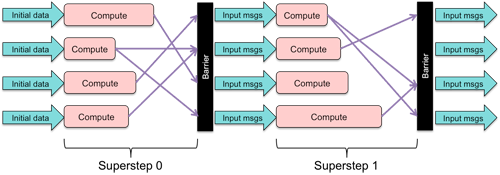
Bulk Synchronous Parallel (BSP) is a programming model and computation framework for parallel computing. Computation is divided into a sequence of supersteps. In each superstep, a collection of processes executes concurrently and creates messages that are sent to other processes. The superstep ends when all the computation in the superstep is complete and all messages are sent. A barrier synchronization at the end of the superstep ensures that all messages have been transmitted (but not yet delivered to the processes). The next superstep begins with the delivery of all those messages to the processes, who then execute their superstep and send messages that will be delivered at the start of the next superstep. This process continues until all processors vote to halt.
Note that no process is permitted to send and receive messages from with another process during a superstep. Any sent messages will be delivered only at the start of the next superstep. This restriction ensures deadlock-free execution.
A popular implementation of the BSP framework is Apache Hama.
Pregel
What’s a graph?
A graph is a set of vertices connected by edges. Edges may be directed from one vertex to another or bidirectional. In computing, a vertex is represented by an object and a directed edge is a link to another object.
Graphs are all around us
They represent computer networks, social groups, roads, disease outbreaks, phone call connections, Twitter followers, Facebook friends, web page links, etc. Some of these graphs have an enormous scale. The world wide web has billions of pages and Facebook has around a billion users.
What’s wrong with MapReduce?
Nothing is wrong with MapReduce. It’s great for many problems. However, many graph traversal problems, when written for MapReduce, end up having to take multiple iterations of MapReduce, with the output of one iteration feeding the input of another. This is not only slow, but it is also inefficient as data has to be written to files at the end of every map and reduce operation and moved between machines. The entire state of the graph needs to be transmitted in each stage, which requires a lot more communication overhead than Pregel, where the vertices and edges remain on the machine that performs the computation. Moreover, MapReduce is not the most direct way of thinking about and logically solving many problems.
Introducing Pregel
Pregel is a software framework created by Google to make it easy to work on huge graphs (e.g., ones with billions of vertices) that span many machines (e.g., tens of thousands). Like MapReduce, the framework relieves the programmer from having to deal with assigning tasks to processors, monitoring jobs, handling failures, and managing communications.
The Pregel framework allows you to write “vertex-centric” code. That is, the same user code, a compute() function, is run concurrently on each vertex of the graph. Each instance of this function keeps track of information, can iterate over outgoing edges (each of which has a value), and can send messages to the vertices connected to those edges or to any other vertices it may know about (e.g., having received a vertex ID via a message).
When a function does not have any more work to do, it votes to halt. This puts the corresponding vertex in an inactive state. When all vertices are in an inactive state, the framework terminates. However, if a vertex’s compute function sends a message to an inactive vertex, that vertex will be made active at the next superstep.
Pregel is an adaptation of the Bulk Synchronous Parallel (BSP) model that is specifically designed with graph processing in mind. Like BSP, Pregel executes in supersteps. Each superstep consists of computation followed by message sending. All messages are synchronized with a barrier, which marks the end of the superstep. At the next superstep, the messages from the previous superstep are delivered to, and available at, the compute function. The downside of using BSP directly for graph processing is that significant additional work would be needed to define, propagate, and maintain the topology (connections) of a graph and map vertices to compute nodes.
Advanced APIs
Since there is overhead in sending messages to vertices, particularly when they are on other machines, Pregel supports the optional use of combiners. A combiner is a user-defined function that is applied to a bunch of messages all targeted to the same vertex. The Combine method processes the values and creates a single input to that vertex. For example, if the goal is to take the sum of a set of values or to choose data from the highest-numbered vertex, the combiner can merge several messages into one before they are transmitted over the network. This does not alter the compute function since it still has to be prepared to receive multiple messages from multiple sources.
To manage global state, such as overall statistics, total edges of a graph, global flags, or minium or maximum values of a vertex, Pregel allows a user to define an aggregator. An aggregator comines received values into one value and makes that value available to all vertices at the next superstep.
Pregel design
Pregel uses a master-slave architecture. Many copies of the program are started on a cluster of machines. One copy becomes the master and is responsible for coordinating activity rather than processing the graph. Others are workers. The master registers itself with a name server (Chubby). Each worker process contacts the name server to find the master. The master assigns one or more sets of vertices to each worker. By default, the assignment is based on the hash of the vertex ID, so neighboring vertices will not necessarily be assigned to the same worker.
The master assigns chunks of input, which is usually resident in GFS or Bigtable, to each worker. Each input item is a set of vertices and its edges. Workers read this input and create either local messages for the vertices they manage or, if the input record is for a remote vertex, send the message to the worker that owns that vertex. All vertices are initially marked as active. The master then asks each worker to perform a superstep. The worker will run concurrent threads, each of which executes a compute function on its vertex. The compute function consumes input, runs its algorithm, and generates zero or more messages to other vertices. Workers send these messages asynchronously but they will not be delivered to their target functions until the next superstep starts. When a worker is done with its processing for one superstep, it informs the master. It also tells the master how many of the vertices it manages will be in the active state in the next superstep. The master waits for all workers to complete before starting the next superstep. This cycle continues until there are no more vertices in the active state.
Fault tolerance
Pregel uses checkpointing for fault tolerance. The master tells each worker to checkpoint itself every N supersteps. Checkpointing means that each vertex has to save its entire state in stable storage. This state will include vertex values, edge values, incoming messages, and possibly any other state that the algorithm needs to track. A master will periodically send ping messages to workers to see if they are alive. If a master does not hear from a worker within a certain window of time, it assumes that the worker has failed. In this case, the master reassigns the set of vertices to other workers and tells all workers to restart their computation from the superstep at the most recent checkpoint.
A popular implementation of Pregel is Apache Giraph, which has been used by Facebook to analyze its social graph.
Spark
Goal: Create a general-purpose high-performance framework for big-data processing.
MapReduce was a powerful framework for parallel computation but it forced a rigid model onto the programmer. Quite often, a computation had to be implemented as a sequence of MapReduce operations. Every map and every reduce operation has to run to completion and write its results to a file before the next sequence of operations can start. Spark creates a highly-flexible framework that lets programmers define their job as a sequence of tranformations and actions on data.
The main client application is called the driver program (or just driver) and is linked with a Spark library. When run, the library creates a SparkContext, which connects to a cluster manager that allocates available worker nodes to run the job. Each worker node runs an executor that contacts the driver for tasks to run. Each executor runs as a process inside a Java Virtual Machine (JVM).
The driver goes through the program, which consists of a sequence of transformations and actions as well as the source data. It creates a directed graph of tasks, identifying how data flows from one transformation to another and ultimately to an action. These tasks are then sent to the executors as jar files for execution. Tasks operate on data and each task is either a transformation or action.
RDDs
Data in Spark is a collection of Resilient Distributed Datasets (RDDs). RDDs can be created in three ways:
They can be data that is a file or set of files in HDFS, key-value data from an Amazon S3 server (similar to Dynamo), HBase data (Hadoop’s version of Bigtable), or the result of a SQL or Cassandra database query.
They can be streaming data obtained using the Spark Streaming connector. As an example, this could be a stream of data from a set of remote sensors.
An RDD can be the output of a transformation function. This is the way one transformation creates data that another transformation will use. To optimize performance, the RDD created by a transfomation is cached in memory (overflowing to disk if necessary).
RDDs have several key properties:
They are immutable. A task can read an RDD and create a new one but cannot modify an existing RDD.
They are typed (structured). An RDD has a structure that a task can parse.
They are partitioned. Parts of an RDD may be sent to different servers. The default partitioning function is to send a row of data to the server corresponding to hash(key) mod servercount.
They are ordered. An RDD contains elements that can be sorted. For example, a list of key-value pairs can be sorted by key. This property is optional.
Transformations and actions
Spark allows two types of operations on RDDs: transformations and actions. Transformations read an RDD and create a new RDD. Example transformations are map, filter, groupByKey, and reduceByKey. Actions are finalizing operations that evaluate an RDD and return a value to the driver program.
Example actions are reduce, grab samples, and write to file.
Lazy evaluation
Spark uses a technique called lazy evaluation for applying transformations. Spark postpones the execution of transformations (operations that modify data) until an action (an operation that triggers a computation to produce results) is called. Instead of executing transformations immediately, Spark constructs a Directed Acyclic Graph (DAG) that identifies the sequence of transformations required for each action. This allows Spark to optimize the processing pipeline, such as combining multiple transformations into a single operation or avoiding running unnecessary transformations, thus reducing computational overhead and improving overall performance.
When an action is requested on an RDD object, the necessary transformations are computed and the result is returned to the driver. Transformations are computed only when some other task needs the RDD that they generate.
Lazy evaluation leads to reduced network and I/O operations, as data is processed only when it is needed.
Fault tolerance
Spark’s lazy evaluation approach aids in efficient fault recovery. In case of a node failure, Spark can recompute only the lost parts of the data using the sequence of transformations, rather than needing to duplicate entire datasets across nodes. This method not only conserves resources but also enhances the robustness and reliability of Spark in handling complex data processing tasks.
For each RDD, the driver tracks, via the DAG, the sequence of transformations that was used to create it. That means an RDD knows which RDDs it is dependent on and which tasks were needed to create each one. If any RDD is lost (e.g., a task that creates one died), the driver can ask the task that generated it to recreate it. The driver maintains the entire dependency graph, so this recreation may end up being a chain of transformation tasks going back to the original data, if necessary.
Kafka publish-subscribe messaging
Goal: Create a high-performance, highly-scalable, reliable message delivery framework.
Kafka messaging is a distributed, fault-tolerant, and high-throughput messaging system that was developed by LinkedIn. It is an open-source project and is widely used in various industries to process and handle large volumes of data in real-time. Kafka is designed to handle data in motion, allowing it to be used in stream processing applications.
Kafka’s architecture is based on the publish-subscribe model. Producers publish messages to Kafka topics, and consumers subscribe to those topics to receive the messages. Kafka brokers act as intermediaries between producers and consumers, storing and distributing messages across the cluster. Brokers can run on a single node or across multiple nodes in a cluster, providing high availability and fault tolerance.
Messages are associated with a category, called a topic. A topic is a way to separate messages based on their content. Each message in Kafka is written to a specific topic, and each topic consists of one or more partitions.
A partition is an ordered, immutable sequence of messages that can be stored on a different Kafka broker in a Kafka cluster (a collection of Kafka servers). The number of partitions for a topic is determined when the topic is created, and can be increased or decreased later.
Partitions allow Kafka to scale horizontally by distributing the data across multiple brokers. Each partition is also replicated across multiple brokers, providing fault tolerance and ensuring that the data is available even if a broker fails. Kafka uses a partitioning scheme to determine which partition a message belongs to based on the key or a custom partitioner. Partitions can also be used to parallelize processing, allowing multiple consumers to process messages from the same topic simultaneously.
Kafka’s replication feature provides fault tolerance by replicating each partition across multiple brokers. When a broker fails, its partitions can be automatically reassigned to other brokers in the cluster, ensuring that the data remains available and that the cluster continues to function normally.
Kafka can be used in queuing or publish-subscribe models. In the queuing model, messages are sent to a single consumer in a first-in-first-out (FIFO) order. In this model, consumers in a consumer group compete for messages in a topic, and each message is only consumed by one consumer. In the publish-subscribe model, messages are available to all subscribed consumers (as long as they are not in the same consumer group). In this model, each message is consumed by all subscribed consumers.
Kafka’s publish-subscribe model allows for flexibility in processing data. Consumers can subscribe to multiple topics, allowing them to process related data from multiple sources. Additionally, Kafka’s ability to partition data allows for parallel processing, allowing multiple consumers to process data from the same topic simultaneously.
Kafka’s queuing model is useful when you need to ensure that each message is only consumed by one consumer. For example, a queue might be used to process orders in an e-commerce system, ensuring that each order is only processed once. Kafka’s queuing model can also be used for tasks like load balancing, where tasks are distributed among multiple consumers.
Content delivery networks
Goal: Provide a highly-scalable infrastructure for caching and serving content from multiple sources.
A content delivery network (CDN) is a set of servers that are usually placed at various points at the edges of the Internet, at various ISPs, to cache content and distribute it to users.
There are several traditional approaches to making a site more scalable and available:
- Proxy servers
- Organizations can pass web requests through caching proxies. This can help a small set of users but you’re out of luck if you are not in that organization.
- Clustering within a datacenter with a load balancer*
- Multiple machines within a datacenter can be load-balanced. However, they all fail if the datacenter loses power or internet connectivity.
- Multihoming
- Machines can be connected with links to multiple networks served by multiple ISPs to guard against ISP failure. However, protocols that drive dynamic routing of IP packets (BGP) are often not quick enough to find new routes, resulting in service disruption.
- Mirroring at multiple sites
- The data can be served at multiple sites, with each machine’s content synchronized with the others. However, synchronization can be difficult.
All these solutions require additional capital costs. You’re building the capability to handle excess capacity and improved availability even if the traffic never comes and the faults never happen.
By serving content that is replicated on a collection of servers, traffic from the main (master) server is reduced. Because some of the caching servers are likely to be closer to the requesting users, network latency is reduced. Because there are multiple servers, traffic is distributed among them. Because all of the servers are unlikely to be down at the same time, availability is increased. Hence, a CDN can provide highly increased performance, scalability, and availability for content.
Akamai
We will focus on one CDN: Akamai. The company evolved from MIT research that was focused on “inventing a better way to deliver Internet content.” A key issue was the flash crowd problem: what if your web site becomes really popular all of a sudden? Chances are, your servers and/or ISP will be saturated and a vast number of people will not be able to access your content. This became known as the slashdot effect.
In late 2022, Akamai ran on 345,000 servers in over 1,300 networks around the world. Around 2015, Akamai served between 15 and 30 percent of all web traffic. Even as several other large-scale content delivery networks emerged and companies such as Google, Amazon, and Apple built their own distribution infrastructure, Akamai still served a peak rate of 250 Tbps of traffic in 2022.
Akamai tries to serve clients from nearest, available servers that are likely to have requested content. According to the company’s statistics, 85% percent of the world’s Internet users are within a single network hop of an Akamai CDN server.
To access a web site, the user’s computer first looks up a domain name via DNS.
A mapping system locates the caching server that can serve the content.
Akamai deploys custom dynamic DNS servers. Customers who use Akamai’s services define their domains as aliases (DNS CNAMEs) that point to an Akamai domain name so that the names will be resolved by these servers.
An Akamai DNS server uses the requestor’s address to find the nearest
edge server that is likely to hold the cached content for the requested site. For example, the domain www.nbc.com
is:
www.nbc.com. 164 IN CNAME www.nbc.com-v2.edgesuite.net.
www.nbc.com-v2.edgesuite.net. 14177 IN CNAME a414.dscq.akamai.net.
or
www.tiktok.com. 271 IN CNAME www.tiktok.com.edgesuite.net.
www.tiktok.com.edgesuite.net. 21264 IN CNAME a2047.api10.akamai.net.
When an Akamai DNS server gets a request to resolve a host name, it chooses the IP address to return based on:
domain name being requested
server health
server load
user location
network status
load balancing
Akamai can also perform load shedding on specific content servers; if servers get too loaded, the DNS server will not respond with those addresses.
Now the user can set up a connection to an Akamai caching server in a cluster of servers in a region at the network edge close to the user and send requests for content. If the content isn’t there, the server broadcasts a query to the other edge servers in that region. If the content isn’t cached at any servers within the region, then the server contacts a parent server. The parent server is also a caching server operated by Akamai but not located at the edge. The goal is to avoid accessing the origin server (the server at the company that hosts the content) unless necessary. If the content isn’t at the parent, the parent queries the other parent servers before giving up and forwarding the request to the origin server via its transport system.
To send data efficiently, Akamai manages an overlay network: the collection of its thousands of servers and statistics about their availability and connectivity. Akamai generates its own map of overall IP network topology based on BGP (Border Gateway Protocol) and traceroute data from various points on the network. It factors in latency and quality of service into its decisions and may choose to route requests to hop through its own servers via always-on TCP links rather than rely on Internet routing, which usually prioritizes the number of hops and cost over speed and quality.
Content servers report their load to a monitoring application. The monitoring application publishes load reports to a local Akamai DNS server, which then determines which IP addresses to return when resolving names.
CDN benefits
A CDN, serving as a caching overlay, provides five distinct benefits:
Caching: static content can be served from caches, thus reducing the load on origin servers.
Routing: by measuring latency, packet loss, and bandwidth throughout its collection of servers, The CDN can find the best route to an origin server, even if that requires forwarding the request through several of its servers instead of relying on IP routers to make the decision.
Security: Because all requests go to the CDN, which can handle a high capacity of requests, it absorbs any Distributed Denial-of-Service attacks (DDoS) rather than overwhelming the origin server. Moreover, any penetration attacks target the machines in the CDN rather than the origin servers. Companies (or a group within a company) that runs the CDN service is singularly focused on the IT infrastructure of the service and is likely to have the expertise to ensure that systems are properly maintained, updated, and are running the best security software. These aspects may be neglected by companies that need to focus on other services.
Analytics: CDNs monitor virtually every aspect of their operation, providing their customers with detailed reports on the quality of service, network latency, and information on where the clients are located.
Cost: CDNs cost money, which is a disadvantage. However, CDNs are an elastic service, which is one that can adapt to changing workloads by using more or fewer computing and storage resources. CDNs provide the ability to scale instantly to surges of demand practically anywhere in the world. They absorb all the traffic so you don’t have to scale up.
Video
Video is the most bandwidth-intensiver service on the Internet and has been a huge source of growth for CDNs. As an example, Netflix operates a global CDN called OpenConnect, which contains up to 80 percent of its entire media catalog. Stored (e.g., on-demand) video is, in some ways, just like any other content that can be distributed and cached. The difference is that, for use cases that require video streaming rather than downloading (i.e., video on demand services), the video stream may be reprocessed, or transcoded, to lower bitrates to support smaller devices or lower-bandwidth connections.
Live video cannot be cached. We have seen the benefits of the fanout that CDNs offer by having many servers at the edge; a video stream can be replicated onto multiple edge servers so it doesn’t have to be sourced from the origin. CDNs can also help with progressive downloads, which is there the user can start watching content while it is still being downloaded.
Today, HTTP Live Streaming, or HLS, is the most popular protocol for streaming video. It allows the use of standard HTTP to deliver content and uses a technique called Adaptive Bitrate Coding (ABR) to deliver video. ABR supports playback on various devices in different formats & bitrates.
The CDN takes on the responsibility of taking the video stream and converting it into to a sequence of chunks. Each chunk represents between 2 and 10 seconds of video. The CDN then encodes each chunk at various bitrates, with can affect the quality & resolution of that chunk of video. For content delivery, the HLS protocol uses feedback from the user’s client to select the optimal chunk. It revises this constantly throughout playback since network conditions can change.
Peer-to-peer content delivery
A peer-to-peer model is an application architecture that removes the need for dedicated servers and enables each host to participate in providing a service. Because all systems can both access as well as provide the service, they are called peers. In this discussion, we will focus on one application: peer-to-peer file distribution via BitTorrent
BitTorrent
The design of BitTorrent was motivated by the flash crowd problem. How do you design a file sharing service that will scale as a huge number of users want to download a specific file? Systems such as Napster, Gnutella, and Kazaa all serve their content from the peer that hosts it. If a large number of users try to download a popular file, all of them will have to share the bandwidth that is available to the peer hosting that content.
The idea behind BitTorrent is to turn each peer that is downloading content into a server of that content. BitTorrent only focuses on the download problem and does not handle the mechanism for locating the content.
To offer content, the content owner creates a .torrent file. This file contains metadata, or information, about the file, such as the name, size of the file, and a SHA-256 hash of the file to allow a downloader to validate its integrity. A peer downloads a file in fixed-size chunks called pieces, and the torrent file also contains the piece size and a list of hashes of each piece of the file. This allows a downloading peer to validate that every downloaded piece has not been modified. Finally, the .torrent file contains the address of a tracker.
The tracker is a server running a process that manages downloads for a set of .torrent files. When a downloading peer opens a .torrent file, it contacts a tracker that is specified in that file. The tracker is responsible for keeping track of which peers have which have all or some pieces of that file. There could be many trackers, each responsible for different torrents.
A seeder is a peer that has the entire file available for download by other peers. Seeders register themselves with trackers so that trackers can direct downloading peers to them. An initial seeder is the initial version of the file.
A leecher is a peer that is downloading files. To start the download, the leecher must have a .torrent file. That identifies the tracker for the contents. It contacts the tracker, which keeps track of the seed nodes that file as well as other leechers, some of whom may have already downloaded some blocks of the file. A leecher contacts seeders and other leechers to download random blocks of the file. As it gets these blocks, it can make them available to other leechers. This allows download bandwidth to scale: every downloader increases overall download capacity. Once a file is fully downloaded, the leecher has the option of turning itself into a seeder and continue to offer serving the file.
Security: Cryptographic Communication
Goal: Use symmetric cryptography, public key cryptography, random numbers, and hash functions to enable exchange encryption keys, provide secure communication, and ensure message.
Security encompasses many things, including authenticating users, hiding data contents while they are at rest (e.g., stored in files) or in motion (moving over a network), destruction of data, masquerading as another server or user, providing false data (e.g., the wrong IP address for a DNS query), and physical premises security. We will focus only on a few topics here.
Security in distributed systems introduces two specific concerns that centralized systems do not have. The first is the use of a network where contents may be seen by other, possibly malicious, parties. The second is the use of servers. Because clients interact with services (applications) running on a server, the application rather than the operating system is responsible for authenticating the client and controlling access to services. Moreover, physical access to the system and the security controls configured for the operating system may be unknown to the client.
Cryptography on its own is not a panacea. Its use will not ensure that a system is secure. However, it is an important component in building secure distributed systems. It is used to implement mechanisms for:
- Confidentiality: ensuring that others cannot read the contents of a message (or file).
- Authentication: proving the origin of a message (or the entity sending that message).
- Integrity: validating that the message has not been modified, either maliciously or accidentally.
- Nonrepudiation: ensuring that senders cannot falsely deny that they authored a message.
Confidentiality
Confidentiality is the classic application of cryptography: obscuring the contents of a message so that it looks like a random bunch of bits that can only be decoded by someone with the proper knowledge. To do this, we start with our plaintext message, P (the term plaintext refers to unencrypted content; it does not need to be text). By applying an encryption algorithm, or cipher, to it, we convert the plaintext to ciphertext, C=E(P). We can decrypt this message to recover the plaintext: P=D(C).
There are two widely-used classes of ciphers: symmetric and public key. A symmetric cipher uses the same to decrypt a message as was used to encrypt it: C=EK(P) and P=DK(C). A public key cipher uses two related keys, known as a key pair. Knowing one key does not enable you to derive the other key of the pair. One of these keys is called the private key because it is never shared. The other key is called the public key because it is freely shared. Examples of popular symmetric encryption algorithms include AES (Advanced Encryption Standard), DES (Data Encryption Standard), and Blowfish.
A message encrypted with one of the keys can only be decrypted with the other key of the pair. For a pair of keys (a, A), where a is the private key and A is the public key, if C=Ea(P) then P=DA(C). A message encrypted with your private key can be decrypted only with your public key. Anyone can do the decryption but you are the only one who could perform the encryption. This becomes the basis for authentication. Conversely, if C=EA(P) then P=Da(C). A message encrypted with your public key can be decrypted only with your private key. Anyone can now perform the encryption but you are the only one can decrypt the message. This becomes the basis for confidentiality and secure communication. Examples of popular public key encryption algorithms include AES (Advanced Encryption Standard), DES (Data Encryption Standard), and Blowfish.
Key distribution
Symmetric encryption algorithms are the dominant means of encrypting files and large streams of data. They are much faster than public key algorithms and key generation is far easier: a key is just a random set of bits rather than two huge numbers with specific properties. The biggest problem with symmetric cryptography is key distribution: ensuring that both sides get the key in a way that no eavesdropper can observe it. For instance, you cannot just send it as a network message containing the key. There are several techniques to handle the problem of key distribution.
Manually, by using pre-shared keys (PSK). This requires setting up the keys ahead of time, such as registering a password or PIN among all the parties that will communicate.
Using a trusted third party. A trusted third party is a trusted server that knows everyone’s keys. If two systems, let’s call them Alice and Bob, want to communicate, the third party will help both of them get the key they need. To communicate, they will use a session key. This is the name for a throw-away key that will be used for one communication session. One way of sharing it is for Alice to create it (it’s just a random number), encrypt it with her secret key, and send it to the trusted third party, which we will name Trent. Since Trent has all the keys, he can decrypt the message with Alice’s secret key to extract the session key. He can then encrypt it for Bob using Bob’s secret key and send it to Bob.
Alternatively, Alice can ask Trent to create a session key that both Alice and Bob will share. Trent will generate the key, encrypt it for Alice, and send it to Alice. He will take the same key, encrypt it for Bob, and send it to Bob. Now they both share a key.
Using the Diffie-Hellman key exchange algorithm. Diiffie-Hellman is a key distribution algorithm, not an encryption algorithm. It uses a one-way function, which is a mathematical function where there is no known way of computing the inverse function to do this. Unfortunately, the algorithm uses the terms public key and private key even though it is not an encryption algorithm; the keys are just numbers, one of which is kept private. Two parties can apply the one-way function to generate a common key that is derived from one public key and one private key. Alice generates a common key that is f(Alice_private_key, Bob_public_key) and Bob generates a common key that is f(Bob_private_key, Alice_public_key). The magic of the mathematics in Diffie-Hellman is that both common keys will be identical and can be used as a symmetric encryption key.
Use public key cryptography. Life becomes easy. If Alice wants to send a message to Bob, she simply encrypts it with Bob’s public key. Only Bob will be able to decrypt it using his private key. If Bob wants to send a message to Alice, he will encrypt it with her public key. Only she will be able to decrypt it using her private key. We have two concerns with this. First, we need to ensure that everyone has trusted copies of public keys (Alice needs to be sure she has Bob’s public key rather than that of an imposter saying he’s Bob). Second, we have to be mindful of the fact that public key cryptography and key generation are both far slower than symmetric cryptography.
To get the best of both worlds, we often rely on hybrid cryptography: we use public key cryptography (often Diffie-Hellman key exchange) only to encrypt a symmetric session key (a small amount of data). From then on, we use symmetric cryptography and encrypt data with that session key.
Message Integrity & Non-repudiation
Without cryptography, we often resort to various error-detecting functions to detect whether there is any data corruption. For example, ethernet uses CRC (cyclic redundancy checksums) and IP headers use 16-bit checksums. A cryptographic hash function is similar in purpose: a function that takes an arbitrary amount of data and generates a fixed set of bits. The hash is much larger than typical checksums – typically 256 or 512 bits – and hence is highly unlikely to result in collisions, where multiple messages result in the same hash value.
A cryptographic hash of a message, H=hash(M) should have the following properties:
It produces a fixed-length output regardless of how long or short the input message is.
It is deterministic; it produces the same results when given the same message as input.
It is a one-way function. Given a hash value H, it should be difficult to figure out what the input is. (N.B.: in cryptography, the term “difficult” means that there are no known shortcuts other that trying every possible input).
It is collision resistant. Given a hash value H, it should be difficult to find another message M’, such that H=hash(M’). Note, however, that because hash values are a finite number of bits in length, multiple messages will hash to the same value (an infinite number, actually). We expect the probability of two messages yielding the same hash value to be so infinitesimally small as to be effectively zero.
Its output should not give any information about any of the input. This is a property of diffusion that applies to encryption algorithms as well. For example, if you notice that anytime the first byte of a message is 0, bit 12 of the hash becomes a 1, the property of diffusion has been violated. Even the smallest change to the message should, on average, alter half the bits of the resulting hash in a seemingly random manner.
It is efficient. We want to be able to generate these easily to validate file contents and messages.
Just augmenting a message with its hash is not sufficient to ensure message integrity. It will allow us to detect that a message was accidentally modified but an attacker who modifies a message can easily recompute a hash function. To prevent the hash from being modified, we can encrypt it.
A message authentication code (MAC) is a hash of a message and a symmetric key. If Alice and Bob share a key, K, Alice can send Bob a message along with a hash concatenated with K. Since Bob also has the key K, he can hash the received message concatenated with K and compare it with the MAC he received from Alice. If an intruder modifies the message, she will not be able to hash it since she will not know K.
A digital signature is similar to a MAC but the hash is encrypted with the sender’s private key. Note that digital signature algorithms are optimized to combine the hashing and encryption but we will describe them as two steps. Here, Alice will send Bob a message along with a hash that is encrypted with her private key. Bob can validate the message and signature by decrypting the signature using Alice’s public key and comparing the result with a hash of the message. A digital signature provides non-repudiation: Alice cannot claim that she did not create the message since only Alice knows her private key. Nobody but Alice could have created the signature. Digital signatures generally use digital signature algorithms that effectively combine the hashing and encryption operations efficiently but can think of signing as a two step process.
We frequently combine covert messaging together with message integrity to ensure that a recipient can detect if a message has been modified. One way of doing this is:
- Alice creates a session key, S (a random number).
- She encrypts the session key with Bob’s public key, B: EB(S) and sends it to Bob.
- Bob decrypts the session key using his private key, b: S = Db(EB(S)). He now knows the session key.
- Alice encrypts the message with the session key, S, using a symmetric algorithm: ES(M).
- Alice creates a signature by encrypting the hash of the message with her private key, a: Ea(H(M)).
- She sends both the encrypted message and signature to Bob.
- Bob decrypts the message using the session key: M = DS(ES(M)).
- Bob decrypts the signature using Alice’s public key A: DA(Ea(H(M)))
- If the decrypted hash matches the hash of the message, H(M), Bob is convinced that the message has not been modified since Alice sent it.
Authentication and Authorization
Goal: Create protocols for authenticating users, establishing secure communication sessions, authorizing services, and passing identities.
Authentication is the process of binding an identity to the user. Note the distinction between authentication and identification. Identification is simply the process of asking you to identify yourself (for example, ask for a login name). Authentication is the process of proving that the identification is correct.
Authorization is when, given an identity, a system decides what access the user is permitted. Authentication is responsible for access control.
Authentication factors
The three factors of authentication are: something you have (such as a key or a card), something you know (such as a password or PIN), and something you are (biometrics).
Each of these factors has pitfalls and can be stolen. Someone can take your access card or see your password. Biometrics are more insidious: they are not precise and if someone can recreate your stolen biometric then you can never use it again.
Combining these factors into a multi-factor authentication scheme can increase security against the chance that any one of the factors is compromised. Multi-factor authentication must use two or more of these factors. Using two passwords, for example, is not sufficient.
Password Authentication Protocol (PAP)
The best-known authentication method is the use of reusable passwords. This is known as the password authentication protocol, or PAP. The system asks you to identify yourself (your login name) and then enter a password. If the password matches that which is associated with the login name on the system then you’re authenticated.
One problem with the protocol is that if someone gets hold of the password file on the system, then they have all the passwords. The common way to thwart this is to store hashes of passwords instead of the passwords themselves. This takes advantage of the one-way property of the hash. To authenticate a user, check if hash(password) = stored_hashed_password. If an intruder gets hold of the password file, they’re still stuck since they won’t be able to reconstruct the original password from the hash. They’ll have to resort to an exhaustive search or a dictionary attack to search for a password that hashes to the value in the file. An exhaustive search may take a prohibitively long time. A dictionary attack is an optimization of the search that does not test every permutation of characters but iterates over common passwords, dictionary words, and common letter-number substitutions.
The other problem with reusable passwords is that if a network is insecure, an eavesdropper may sniff the password from the network. A potential intruder may also simply observe the user typing a password. To thwart this, we can turn to one-time passwords. If someone sees you type your credentials or read them from the network stream, it won’t matter because that information will be useless for future logins.
CHAP Authentication
The Challenge-Handshake Authentication Protocol (CHAP) is an authentication protocol that allows a server to authenticate a user without sending a password over the network.
Both the client and server share a secret (such as a password). A server creates a random bunch of bits (called a nonce) and sends it to the client (user) that wants to authenticate. This is the challenge.
The client identifies itself and sends a response that is the hash of the shared secret combined with the challenge. The server has the same data and can generate its own hash of the same challenge and secret. If the hash matches the one received from the client, the server is convinced that the client knows the shared secret and is therefore legitimate.
An intruder who sees this hash cannot extract the original data. An intruder who sees the challenge cannot create a suitable hashed response without knowing the secret. Note that this technique requires passwords to be accessible at the
server and the security rests on the password file remaining secure.
Time-based: TOTP
With the Time-based One Time Password (TOTP) protocol, both sides share a secret key. To authenticate, a user runs the TOTP function to create a one-time password. The TOTP function is a password created as a hash of a shared secret key and the time of day. The service, who also knows the secret key and time, can generate the same hash and thus validate the value presented by the user.
TOTP is often used as a second factor (proof that you have some device with the secret configured in it) in addition to a password. The protocol is widely supported by companies such as Amazon, Dropbox, Wordpress, Microsoft, and Google.
Public key authentication
Public key authentication relies on the use of nonces. A nonce is simply a randomly-generated bunch of bits and is sent to the other party as a challenge for them to prove that they are capable of encrypting something with a specific key that they possess. The use of a nonce is central to public key authentication.
If Alice wants to authenticate Bob, she needs to have Bob prove that he possesses his private key (private keys are never shared). To do this, Alice generates a nonce (a random bunch of bits) and sends it to Bob, asking him to encrypt it with his private key. If she can decrypt Bob’s response using Bob’s public key and sees the same nonce, she will be convinced that she is talking to Bob because nobody else will have Bob’s private key. Mutual authentication requires that each party authenticate itself to the other: Bob will also have to generate a nonce and ask Alice to encrypt it with her private key.
Passkey authentication is an implementation of public key authentication that is designed to eliminate the use of passwords. A user first logs onto a service via whatever legacy login protocol the service supports: typically a username-password or the additional use of a time-based one-time password or SMS authentication code. After that, the user’s device generates a public-private key pair for that specific service. The public key is sent to the service and associated with the user, much like a passwword was in the past. Note that the public key is not secret. The private key is stored on the user’s device.
Once passkey authentication is set up, the user logs in by providing their user name. The server generates a random challenge string (at least 16 bytes long) and sends it to the user. The user’s device retrieves the private key for the desired service. This is generally stored securely on the device and unlocked via Face ID, Touch ID, or a local password. None of this information, including the private key, is sent to the server. Using the private key, the device creates a digital signature for the challenge provided by the service and sends the result to server. The server looks up the user’s public key, which was registered during enrollment, and verifies the signature against the challenge (that is, decrypt the data sent by the user and see if it matches a hash of the original challenge string). If the signature is valid, the service is convinced that the other side holds a valid private key that corresponds to the public key that is associated with the user and is therefore the legitimate user.
Identity binding: digital certificates
While public keys provide a mechanism for asserting integrity via digital signatures, they are themselves anonymous. We’ve discussed a scenario where Alice uses Bob’s public key but never explained how she can be confident that the key really belongs to Bob and was not planted by an adversary. Some form of identity binding of the public key must be implemented for you to know that you really have my public key instead of someone else’s.
Digital certificates provide a way to do this. A certificate is a data structure that contains user information (called a distinguished name) and the user’s public key. To ensure that nobody changes any of this data, this data structure also contains a signature of the certification authority. The signature is created by taking a hash of the rest of the data in the structure and encrypting it with the private key of the certification authority. The certification authority (CA) is an organization that is responsible for setting policies of how they validate the identity of the person who presents the public key for encapsulation in a certificate.
To validate a certificate, you hash all the certificate data except for the signature. Then you would decrypt the signature using the public key of the issuer. If the two values match, then you know that the certificate data has not been modified since it has been signed. The challenge now is how to get the public key of the issuer. Public keys are stored in certificates, so the issuer would also have a certificate containing its public key. The certificates for many of the CAs are preloaded into operating systems or, in some cases, browsers.
Transport Layer Security (TLS)
Secure Sockets Layer (SSL) was created as a layer of software above TCP that provides authentication, integrity, and encrypted communication while preserving the abstraction of a sockets interface to applications. An application sets up an SSL session to a service. After that, it simply sends and receives data over a socket just like it would with the normal sockets-based API that operating systems provide. The programmer does not have to think about network security. As SSL evolved, it morphed into a new version called TLS, Transport Layer Security. While SSL is commonly used in conversation, all current implementations are TLS.
Any TCP-based application that may not have addressed network security can be security-enhanced by simply using TLS. For example, the standard email protocols, SMTP, POP, and IMAP, all have TLS-secured interfaces. Web browsers use HTTP, the Hypertext Transfer Protocol, and also support HTTPS, which is the exact same protocol but uses A TLS connection.
TLS provides:
- Authentication
- TLS provides allows endpoints to authenticate prior to sending data. It uses public key authentication via X.509 certificates. Authentication is optional and can be unidirectional (the client may just authenticate the server), unidirectional (each side authenticates the other), or none (in which case we just exchange keys but do not validate identities).
- Key exchange
- After authentication, TLS performs a key exchange so that both sides can obtain random shared session keys.
- Data encryption
- Symmetric cryptography is used to encrypt data.
- Data integrity
- Ensure that we can detect if data in transit has not been modified. TLS includes a MAC with transmitted data.
- Interoperability & evolution
- TLS was designed to support many different key exchange, encryption, integrity, & authentication protocols. The start of each session enables the protocol to negotiate what protocols to use for the session.
Service Authorization via OAuth
Goal: Enable users to provide limited permissions for one service to access another service.
Suppose that you want an app (or some network service) to access your Google calendar. One way to do this is to provide the app with the ID and password of your Google account. Unfortunately, this gives the app full control of the entire account and is something you may not feel comfortable granting.
OAuth is designed to allow users to control what data or services one service can access from another service.
For example, if you want a photo printing service to access photos from your flickr account, you don’t want to provide it with your flickr username and password for unrestricted access. Instead, OAuth allows you to specify what specific access you allow the photo printing service to have to your flickr account and for how long. Token credentials (a bunch of bits as far as your app or website is concerned) are used in place of the resource owner’s username and password for gaining access to the service. These token credentials include a token identifier and a secret.
There are three entities involved in OAuth:
User: the user and the user’s browser.
Client (application, called the consumer): this is the service that the user is accessing. For example, the moo.com photo printing service
Authorization Server, acting as the Service Provider (server, also called the provider): this is the service that the consumer needs to access. For example, flicker.com to get the photos
We will use the moo.com photo printing and the flickr.com photo serving service as an example.
Alice wants to order some prints and logs into moo.com. Moo knows about flickr and allows her to select select flickr as the source of her photos. When Moo built its service, its developers registered the service with flickr and obtained OAuth client credentials (client ID and secret).
When Alice selects flickr, moo.com needs to contact flickr.com for an authorization code, which is a temporary credential. The application, moo.com, creates a request that contains a scope: a list of requested services that it needs from flickr (for example, get photos from your account). The application then redirects Alice to to the flickr OAuth page (that could be a separate server at flickr) with a directive to redirect her back to Moo when she’s done. The redirect request to Flickr contains the app’s ID, the app’s secret, and the scope.
At the flickr OAuth page, she authenticates (using whatever authentication mechanism the service requires, such as login/password or perhaps via OpenID, which may cause another level of redirection, depending on how the service handles authentication) and is presented with a consent form. This is a description of what moo.com is requesting to do (e.g., access to download photos for the next 10 minutes). She can approve or reject the request.
When Alice approves the request, she is redirected back to moo.com. The redirect contains a one-time-use authorization code.
Moo now contacts flickr.com directly and exchanges the authorization code for an access token. Authorization codes are used to obtain a user’s approval. Since they are sent back via an HTTP REDIRECT, they may not be sent securely but the Service Provider will only accept them from the client to which they were issued. Access tokens are used to access resources on the provider (server); that is, to call APIs.
Moo now sends API requests to flickr to download the requested photos. Every request must include the access token, which the service provider (flickr) will validate each time to ensure that the user has not revoked the ability of the client to access these services.
Distributed Authentication via OpenID Connect
Goal: Allow services to use a third-party user authentication service to authenticate a user.
OpenID Connect was created to solve the problem of alleviating a user from managing multiple identities (logins and passwords) at many different services (web sites).
It is not an authentication protocol. Rather, it’s a technique for a service to redirect authentication to a specific OpenID Connect authentication server and have that server be responsible for authentication.
OpenID Connect is an identity layer on top of the OAuth 2.0 protocol. It uses the basic protocol of OAuth and the same communication mechanisms: HTTPS with JSON messages.
There are three entities involved:
Client: this is the application that the user is accessing. It is often a web site but may be a dedicated app. (In OAuth, we think of this as the consumer).
User: this is the user’s browser. In the case of a dedicated app, the app will take this role.
Authorization Server, acting as the Identity Provider. This server is responsible for managing your ID and authenticating you. It is called the Authorization Server because OpenID Connect is built on top of OAuth 2.0, a service authorization framework. For OAuth, we refer to this as a Service Provider instead of an Identity Provider.
OpenID Connect allows a client to answer the question, what is the identity of the person using this service?
The protocol takes the same steps as OAuth; it redirects the user to the Identity Provider, which authenticates the user and redirects the user back to the client app with a one-time authorization code. The client then contacts the Identity Provider for an access token.
Here’s the basic flow:
Before OpenID Connect is used, a client may pre-register its service with an Identity Provider’s Authorization Server. This allows the Identity Provider administrator to configure policies that set restrictions on accessing user information. It also allows a client and server to agree upon a shared secret that will be used to validate future messages. If this is not done, public key cryptography can be used to transmit a key to establish a secure channel between the client and the Identity Provider.
The user is presented with a request to log in. The client may choose to support multiple Identity Providers. In this case, the user identifies the Identity Provider in the user name. For example,
joe@example.com
. The protocol then knows that the OpenID Connect Authorization Server is located atexample.com
. Alternatively, the provider may require the use of a specific provider (e.g., Google, Microsoft). The client redirects the user to the Authorization Server at the Identity Provider via an HTTP REDIRECT message.The redirect results in the client sending an Authentication Request to the Identity Provider. This is an OAuth message with a scope (access request) that requests "
openid
'. The scope may also include a request for other identifying data, such as a user profile, email, postal address, and phone number.The Identity Provider (Authorization Server) authenticates the user using whatever protocol it chooses to use. That decision is left up to the administrators of the Authorization Server.
After authenticating the user, the Identity Provider requests the user’s permission for all the requests listed in the scope. For example, SomeApp requests permission for: signing you in, your profile, your email address, your address, your phone number. This is the same consent form as a user will see with any OAuth request for services.
If the user approves, the Identity Provider sends a redirect to send the browser back to the client. This redirect message contains an authorization code created by the Identity Provider that is now given to the client. The authorization code looks like a large bunch of random text and has no meaning to the user or the client.
The client now sends a token request directly to the Identity Provider. The request includes the authorization code that it received. All traffic is encrypted via HTTPS to guard against eavesdroppers. If the client pre-registered, the request will contain the pre-established secret so that the Authorization Server can validate the client.
The server returns an access token along with an ID token to the client. Note that the user is not involved in this flow.
The ID token asserts the user’s identity. It is a JSON object that:
- Identifies the Identity Provider (Authorization Server)
- Has an issue and expiration date
- May contain additional details about the user or service
- Is digitally signed by the Identity Provider using either the provider’s private key or a shared secret that was set up during registration.
By getting this ID token, the client knows that, as far as the Identity Provider is concerned, the user has successfully authenticated. At this point, the client does not need to contact the Identity Provider anymore.
The access token is the same access token that OAuth provides clients so that they can request services. It has an expiration date associated with it and may be passed to the Identity Provider to request access to detailed information about the user or get other protected resources. What the client can request is limited by the scope of the Authentication Request in step 2.
OpenID and OAuth were separate protocols until 2014. They were then merged so that OpenID Connect is a special mode of OAuth that requests authentication and provides the client with an ID token. However, the purpose of OAuth and OpennID are fundamentally different. OpenID is designed to allow a third party to manage user authentication. That is, it provides single sign-on: allowing a user to use the same login and a third party (OpenID) authentication service for many web sites. OAuth, on the other hand, is designed for service authorization. It is up to the remote service you are accessing to decide if and how to authenticate you before allowing you to authorize access to that service.